February 7, 2023 Tuesday
Abstract:
The field of computer vision has seen significant advancements in recent years, resulting in a range of practical applications such as virtual and augmented reality, and self-driving cars. However, the reliance on costly human annotations can be a hindrance in complex and novel vision tasks. In this talk, I will discuss ways to achieve detailed visual recognition (e.g. semantic part segmentation and 3D pose estimation) with minimal human supervision through techniques such as self-supervised learning, utilizing weak supervision, and cross-modal learning. These methods not only address core computer vision challenges but also open up new opportunities in ecology and 3D content creation.
Speaker Bio:
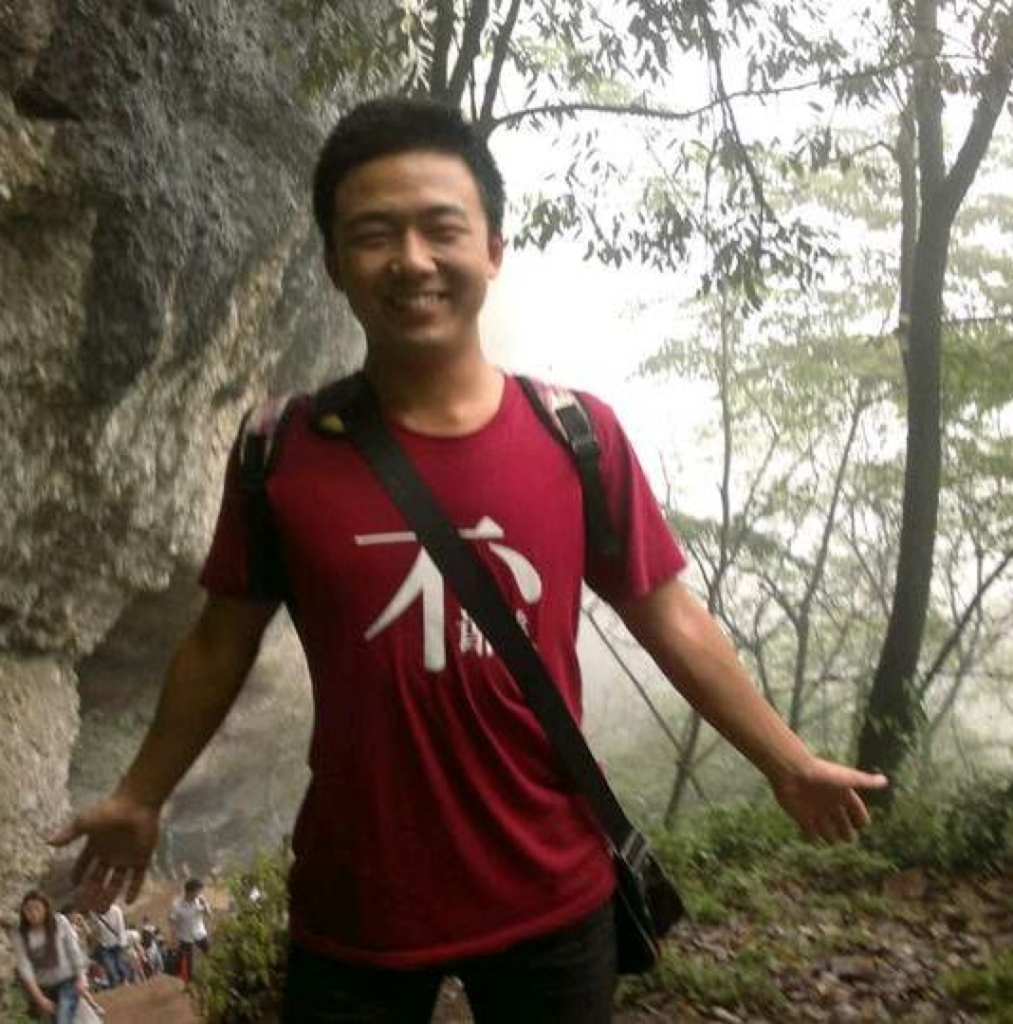
Zezhou CHENG
PhD Candidate, Computer Science at the University of Massachusetts Amherst
Zezhou Cheng is a Ph.D. candidate in Computer Science at the University of Massachusetts Amherst, where he is advised by Prof. Subhransu Maji. His research interests include computer vision, machine learning, and their applications to ecology, virtual reality/augmented reality, and autonomous vehicles. His current research focuses on developing techniques for 3D scene understanding and generation with minimal human supervision. He has received awards for his work, such as the Best Synthesis Award in the Computer Science Department at the University of Massachusetts Amherst in 2020 and the Best Poster Award at the New England Computer Vision Workshop in 2019. He has also served on the program committee at major computer vision conferences and was recognized as an Outstanding Reviewer at CVPR 2020. During his studies, he interned at Google Research, Snap Research, and Amazon. In addition, he has been collaborating with ecologists from Cornell Lab of Ornithology and Colorado State University to tackle challenges in animal conservation with machine learning techniques.
Personal Website: https://sites.google.com/site/zezhoucheng/