10月2日至10月6日,计算机视觉国际会议(ICCV 2023)在巴黎会议中心举办。香港科技大学(广州)信息枢纽共有10项成果获录用,其中人工智能学域博士生葛汶杭获最佳论文提名奖,并在会议中进行了口头报告(口头报告接受率为1.8%),其论文“Ref-NeuS: Ambiguity-Reduced Neural Implicit Surface Learning for Multi-View Reconstruction with Reflection”被程序委员会选为最佳论文候选名单(共17篇论文选为最佳论文候选名单,接受率为0.2%)。
· ICCV 2023
ICCV成立于1987年,自那以后每两年举办一次,会议上将颁发最佳论文奖(Marr Prize)和最佳论文提名奖。它已经成为计算机视觉领域最具影响力的顶级会议之一,并在全球范围内吸引了众多研究人员和专家的参与。这个会议通常涵盖图像处理、模式识别、计算机视觉算法、机器学习以及与计算机视觉相关的其他主题。ICCV推动了计算机视觉研究和应用的发展,并为研究人员提供了一个交流和合作的重要平台。

在本次ICCV会议中,香港科技大学(广州)人工智能学域的博士生葛汶杭围绕利用隐式表面表示以及体素渲染对反光物体进行多视角重建的问题,进行了口头报告。该研究为港科大(广州)与思谋科技合作完成,提出多视角一致性是重建物体的先决条件,而反光物体与环境光的交互破坏了这种一致性,从而导致重建的误差。为了解决这个问题,该论文利用基于投影的多视图信息,将反光视图建模为异常样本,提出了反光得分来表示异常值。在建模光度一致性损失函数的时候,利用估计的反光得分对光度一致性损失进行重权重,以削弱反光异常值对重建的影响。此项研究帮助我们更好地重建反光物体,对后续的任务例如3D打印,AR,VR提供重要的应用价值。
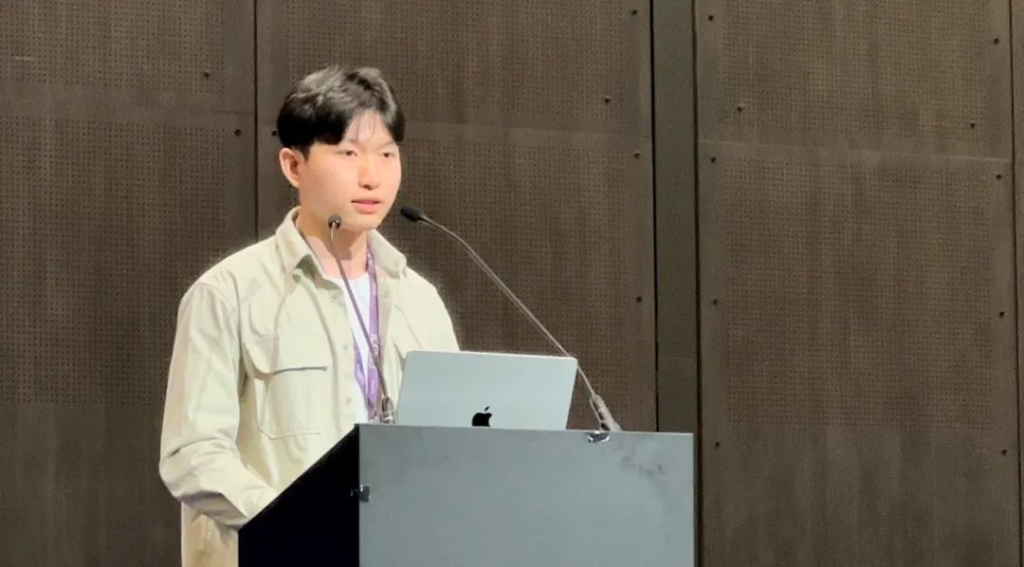
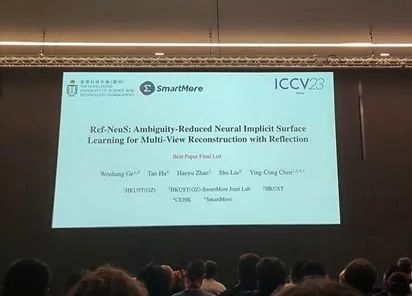
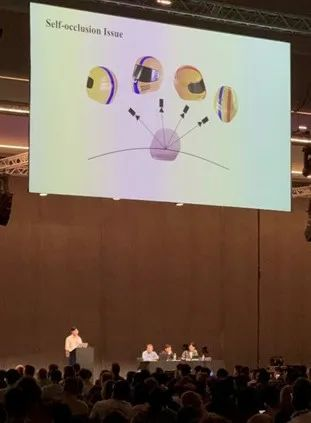
此次作学术报告的葛汶杭在人工智能学域助理教授陈颖聪的指导下攻读人工智能方向的博士学位。陈颖聪教授的研究团队重点关注计算机视觉中的生成模型,一方面研究扩散模型在数据生成编辑上的应用,另一方面研究三维重建与渲染以及3D生成。在ICCV 2023会议上,陈颖聪教授团队共在生成模型主题上发表三篇文章。

在报告的最后,葛汶杭向所有参会者介绍了香港科技大学(广州),并热情邀请来自全世界的研究人员到香港科技大学(广州)访问学习,一起在人工智能领域探讨与合作。
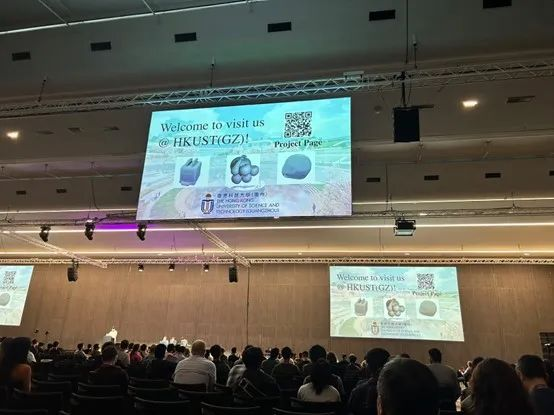
–
在本届ICCV会议上,信息枢纽的师生们成果瞩目,共有10篇论文被录用发表:
· Ref-NeuS: Ambiguity-Reduced Neural Implicit Surface Learning for Multi-View Reconstruction with Reflection
Wenhang Ge, Tao Hu, Haoyu Zhao, Shu Liu, Ying-Cong Chen
Abstract: Neural implicit surface learning has shown significant progress in multi-view 3D reconstruction, where an object is represented by multilayer perceptrons that provide continuous implicit surface representation and view-dependent radiance. However, current methods often fail to accurately reconstruct reflective surfaces, leading to severe ambiguity. To overcome this issue, we propose Ref-NeuS, which aims to reduce ambiguity by attenuating the effect of reflective surfaces. Specifically, we utilize an anomaly detector to estimate an explicit reflection score with the guidance of multiview context to localize reflective surfaces. Afterward, we design a reflection-aware photometric loss that adaptively reduces ambiguity by modeling rendered color as a Gaussian distribution, with the reflection score representing the variance. We show that together with a reflection direction dependent radiance, our model achieves high-quality surface reconstruction on reflective surfaces and outperforms the state-of-the-arts by a large margin. Besides, our model is also comparable on general surfaces.
–
· Not All Steps are Created Equal: Selective Diffusion Distillation for Image Manipulation
Luozhou Wang, Shuai Yang, Shu Liu, Ying-Cong Chen
Abstract: Conditional diffusion models have demonstrated impressive performance in image manipulation tasks. The general pipeline involves adding noise to the image and then denoising it. However, this method faces a trade-off problem: adding too much noise affects the fidelity of the image while adding too little affects its editability. This largely limits their practical applicability. In this paper, we propose a novel framework, Selective Diffusion Distillation (SDD), that ensures both the fidelity and editability of images. Instead of directly editing images with a diffusion model, we train a feedforward image manipulation network under the guidance of the diffusion model. Besides, we propose an effective indicator to select the semantic-related timestep to obtain the correct semantic guidance from the diffusion model. This approach successfully avoids the dilemma caused by the diffusion process. Our extensive experiments demonstrate the advantages of our framework. Code is released at https://github.com/AndysonYs/Selective-Diffusion[1]Distillation.
–
· Out-of-domain GAN inversion via Invertibility Decomposition for Photo-Realistic Human Face Manipulation
Xin Yang, Xiaogang Xu, Ying-Cong Chen
Abstract: The fidelity of Generative Adversarial Networks (GAN) inversion is impeded by Out-Of-Domain (OOD) areas (e.g., background, accessories) in the image. Detecting the OOD areas beyond the generation ability of the pre-trained model and blending these regions with the input image can enhance fidelity. The “invertibility mask” figures out these OOD areas, and existing methods predict the mask with the reconstruction error. However, the estimated mask is usually inaccurate due to the influence of the reconstruction error in the In-Domain (ID) area. In this paper, we propose a novel framework that enhances the fidelity of human face inversion by designing a new module to decompose the input images to ID and OOD partitions with invertibility masks. Unlike previous works, our invertibility detector is simultaneously learned with a spatial alignment module. We iteratively align the generated features to the input geometry and reduce the reconstruction error in the ID regions. Thus, the OOD areas are more distinguishable and can be precisely predicted. Then, we improve the fidelity of our results by blending the OOD areas from the input image with the ID GAN inversion results. Our method produces photo[1]realistic results for real-world human face image inversion and manipulation. Extensive experiments demonstrate our method’s superiority over existing methods in the quality of GAN inversion and attribute manipulation. Our code is available at: AbnerVictor/OOD-GAN-inversion.
–
· Dynamic PlenOctree for Adaptive Sampling Refinement in Explicit NeRF
Haotian Bai, Yiqi Lin, Yize Chen, Lin Wang
Abstract: The explicit neural radiance field (NeRF) has gained considerable interest for its efficient training and fast inference capabilities, making it a promising direction such as virtual reality and gaming. In particular, PlenOctree (POT) , an explicit hierarchical multi-scale octree representation, has emerged as a structural and influential framework. However, POT’s fixed structure for direct optimization is sub-optimal as the scene complexity evolves continuously with updates to cached color and density, necessitating refining the sampling distribution to capture signal complexity accordingly. To address this issue, we propose the dynamic PlenOctree (DOT), which adaptively refines the sample distribution to adjust to changing scene complexity. Specifically, DOT proposes a concise yet novel hierarchical feature fusion strategy during the iterative rendering process. Firstly, it identifies the regions of interest through training signals to ensure adaptive and efficient refinement. Next, rather than directly filtering out valueless nodes, DOT introduces the sampling and pruning operations for octrees to aggregate features, enabling rapid parameter learning. Compared with POT, our DOT outperforms it by enhancing visual quality, reducing over 55.15/68.84% parameters, and providing 1.7/1.9 times FPS for NeRF-synthetic and Tanks & Temples, respectively.
[1] Yu, Alex, et al. “Plenoctrees for real-time rendering of neural radiance fields.” Proceedings of the IEEE/CVF International Conference on Computer Vision. 2021
https://arxiv.org/abs/2307.15333
–
· OmniZoomer: Learning to Move and Zoom in on Sphere at High-Resolution
Zidong Cao, Hao Ai, Yan-Pei Cao, Ying Shan, Xiaohu Qie, Lin Wang
Abstract: Omnidirectional images (ODIs) have become increasingly popular, as their large field-of-view (FoV) can offer viewers the chance to freely choose the view directions in immersive environments such as virtual reality. The Mobius transformation is typically employed to further provide the opportunity for movement and zoom on ODIs, but applying it to the image level often results in blurry effect and aliasing problem. In this paper, we propose a novel deep learning-based approach, called OmniZoomer, to incorporate the Mobius transformation into the network for movement and zoom on ODIs. By learning various transformed feature maps under different conditions, the network is enhanced to handle the increasing edge curvatures, which alleviates the blurry effect. Moreover, to address the aliasing problem, we propose two key components. Firstly, to compensate for the lack of pixels for describing curves, we enhance the feature maps in the high-resolution (HR) space and calculate the transformed index map with a spatial index generation module. Secondly, considering that ODIs are inherently represented in the spherical space, we propose a spherical resampling module that combines the index map and HR feature maps to transform the feature maps for better spherical correlation. The transformed feature maps are decoded to output a zoomed ODI. Experiments show that our method can produce HR and high-quality ODIs with the flexibility to move and zoom in to the object of interest. Project page is available at http://vlislab22.github.io/OmniZoomer/
–
· Look at the Neighbor: Distortion-aware Unsupervised Domain Adaptation for Panoramic Semantic Segmentation
Xu Zheng, Tianbo, Yunhao Luo, Lin Wang
Abstract: Endeavors have been recently made to transfer knowledge from the labeled pinhole image domain to the unlabeled panoramic image domain via Unsupervised Domain Adaptation (UDA). The aim is to tackle the domain gaps caused by the style disparities and distortion problem from the non-uniformly distributed pixels of equirectangular projection (ERP). Previous works typically focus on transferring knowledge based on geometric priors with specially designed multi-branch network architectures. As a result, considerable computational costs are induced, and meanwhile, their generalization abilities are profoundly hindered by the variation of distortion among pixels. In this paper, we find that the pixels’ neighborhood regions of the ERP indeed introduce less distortion. Intuitively, we propose a novel UDA framework that can effectively address the distortion problems for panoramic semantic segmentation. In comparison, our method is simpler, easier to implement, and more computationally efficient. Specifically, we propose distortion-aware attention (DA) capturing the neighboring pixel distribution without using any geometric constraints. Moreover, we propose a class-wise feature aggregation (CFA) module to iteratively update the feature representations with a memory bank. As such, the feature similarity between two domains can be consistently optimized. Extensive experiments show that our method achieves new state-of-the-art performance while remarkably reducing 80% parameters.
–
· A Good Student is Cooperative and Reliable: CNN-Transformer Collaborative Learning for Semantic Segmentation
Jinjing Zhu, Yunhao Luo, Xu Zheng, Hao Wang, Lin Wang
Abstract: In this paper, we strive to answer the question ‘how to collaboratively learn convolutional neural network (CNN)- based and vision transformer (ViT)-based models by selecting and exchanging the reliable knowledge between them for semantic segmentation?’ Accordingly, we propose an online knowledge distillation (KD) framework that can simultaneously learn compact yet effective CNN-based and ViT-based models with two key technical breakthroughs to take full advantage of CNNs and ViT while compensating their limitations. Firstly, we propose heterogeneous feature distillation (HFD) to improve students’ consistency in low-layer feature space by mimicking heterogeneous features between CNNs and ViT. Secondly, to facilitate the two students to learn reliable knowledge from each other, we propose bidirectional selective distillation (BSD) that can dynamically transfer selective knowledge. This is achieved by 1) region-wise BSD determining the directions of knowledge transferred between the corresponding regions in the feature space and 2) pixel-wise BSD discerning which of the prediction knowledge to be transferred in the logit space. Extensive experiments on three benchmark datasets demonstrate that our proposed framework outperforms the state-of-the-art online distillation methods by a large margin, and shows its efficacy in learning collaboratively between ViT based and CNN-based models.
–
· Benchmarking and Analyzing Robust Point Cloud Recognition: Bag of Tricks for Defending Adversarial Examples
Qiufan Ji, Lin Wang, Cong Shi, Shengshan Hu, Yingying Chen, Lichao Sun
Abstract: Deep Neural Networks (DNNs) for 3D point cloud recognition are vulnerable to adversarial examples, threatening their practical deployment. Despite the many research endeavors have been made to tackle this issue in recent years, the diversity of adversarial examples on 3D point clouds makes them more challenging to defend against than those on 2D images. For examples, attackers can generate adversarial examples by adding, shifting, or removing points. Consequently, existing defense strategies are hard to counter unseen point cloud adversarial examples. In this paper, we first establish a comprehensive, and rigorous point cloud adversarial robustness benchmark to evaluate adversarial robustness, which can provide a detailed understanding of the effects of the defense and attack methods. We then collect existing defense tricks in point cloud adversarial defenses and then perform extensive and systematic experiments to identify an effective combination of these tricks. Furthermore, we propose a hybrid training augmentation methods that consider various types of point cloud adversarial examples to adversarial training, significantly improving the adversarial robustness. By combining these tricks, we construct a more robust defense framework achieving an average accuracy of 83.45% against various attacks, demonstrating its capability to enabling robust learners. Our codebase are open-sourced on: https://github.com/ qiufan319/benchmark_pc_attack.git.
–
· Global Balanced Experts for Federated Long-Tailed Learning
Yaopei Zeng, Lei Liu, Li Liu, Li Shen, Shaoguo Liu, Baoyuan Wu
Abstract: Federated learning (FL) is a prevalent distributed machine learning approach that enables collaborative training of a global model across multiple devices without sharing local data. However, the presence of long-tailed data can negatively deteriorate the model’s performance in real-world FL applications. Moreover, existing re-balance strategies are less effective for the federated long-tailed issue when directly utilizing local label distribution as the class prior at the clients’ side. To this end, we propose a novel Global Balanced Multi-Expert (GBME) framework to optimize a balanced global objective, which does not require additional information beyond the standard FL pipeline. In particular, a proxy is derived from the accumulated gradients uploaded by the clients after local training, and is shared by all clients as the class prior for re-balance training. Such a proxy can also guide the client grouping to train a multi-expert model, where the knowledge from different clients can be aggregated via the ensemble of different experts corresponding to different client groups. To further strengthen the privacy-preserving ability, we present a GBME-p algorithm with a theoretical guarantee to prevent privacy leakage from the proxy. Extensive experiments on long-tailed decentralized datasets demonstrate the effectiveness of GBME and GBME-p, both of which show superior performance to state-of-the-art methods. The code is available at here.
–
· Synchronize Feature Extracting and Matching: A Single Branch Framework for 3D Object Tracking
Teli Ma, Mengmeng Wang, Jimin Xiao, Huifeng Wu, Yong Liu
Abstract: Siamese network has been a de facto benchmark framework for 3D LiDAR object tracking with a shared-parametric encoder extracting features from template and search region, respectively. This paradigm relies heavily on an additional matching network to model the cross-correlation/similarity of the template and search region. In this paper, we forsake the conventional Siamese paradigm and propose a novel single-branch framework, SyncTrack, synchronizing the feature extracting and matching to avoid forwarding encoder twice for template and search region as well as introducing extra parameters of matching network. The synchronization mechanism is based on the dynamic affinity of the Transformer, and an in-depth analysis of the relevance is provided theoretically. Moreover, based on the synchronization, we introduce a novel Attentive Points-Sampling strategy into the Transformer layers (APST), replacing the random/Farthest Points Sampling (FPS) method with sampling under the supervision of attentive relations between the template and search region. It implies connecting point-wise sampling with the feature learning, beneficial to aggregating more distinctive and geometric features for tracking with sparse points. Extensive experiments on two benchmark datasets (KITTI and NuScenes) show that SyncTrack achieves state-of-the-art performance in real-time tracking.
–