Feb 22, 2024 Thursday
Abstract:
In recent years, transfer learning from pre-trained models has become a common practice in low-resource computer vision and natural language processing applications. As transfer performance is largely affected by the choice of the source task or model, where an inappropriate match could lead to “negative transfer”, an efficient, easy-to-compute metric for model transferability is critical. In this talk, we will introduce the problem of transferability estimation, highlighting representative metrics. Furthermore, we will show how optimizing transferability can lead to better target performance for various transfer learning paradigms, such as model fine-tuning, and domain generalization.
Time:
Feb 22, 2024 Thursday
11:00-11:50
Location:
Rm W1-101, GZ Campus
Online Zoom
Join Zoom at: https://hkust-gz-edu-cn.zoom.us/j/4236852791 OR 423 685 2791
Speaker Bio:
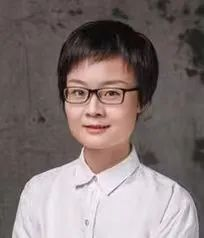
李阳, Yang LI
Associate Professor
Tsinghua-Berkeley Shenzhen Institute, Tsinghua Shenzhen International School
Yang Li received her B.A. degree and PhD mathematics and computer science from Smith College in 2011, and the Ph.D. degree in computer science from Stanford University in 2017. She has joined Tsinghua-Berkeley Shenzhen Institute, Tsinghua Shenzhen International School since 2017, currently working as an Associate Professor, and a principal investigator in the Shenzhen Key Laboratory of Ubiquitous Data Enabling. She is interested in transfer learning, representation learning for multiple tasks and domains, spatial and topological data analysis. Her recent works also focus on optimizing model selection and knowledge transfer strategies for medical image understanding. She is a member of IEEE, ACM and an associate editor of Franklin Open and an editorial board member of Digital Signal Processing.