Apr 11, 2024 Thursday
Abstract:
The learning paradigm of deep neural networks has undergone a significant shift in recent years. Traditional deep learning approaches have been complemented by emerging learning from model techniques such as transferring knowledge, editing models, fusing models, or leveraging unlabeled data to tune models, among which deep model fusion technique has shown promise in improving performance, accelerating training, and reducing the need for labeled data. However, there are still challenges in effectively fusing models and scaling these techniques to large foundation models. In this talk, we systematically investigate model fusion techniques in deep neural networks. We introduce the background, motivation, and applications of these techniques. Additionally, we offer a brief overview of existing model fusion techniques and propose a taxonomy to categorize them. Furthermore, we highlight some of our recent work on model fusion techniques, including model fusion via graph matching alignment, model fusion via learnable adaptive weights, model fusion via subspace learning, and enhanced multi-task model fusion with pre- and post-finetuning by reducing representation bias between the merged model and individual task. Finally, we discuss the challenges and future directions in model fusion techniques.
Time:
Apr 11, 2024 Thursday
11:00-11:50
Location:
Rm W1-101, GZ Campus
Online Zoom
Join Zoom at: https://hkust-gz-edu-cn.zoom.us/j/4236852791 OR 423 685 2791
Speaker Bio:
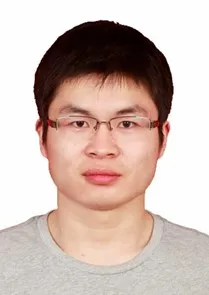
Dr. Li SHEN
Research Scientist, JD Explore Academy, Beijing,China
Li Shen is currently a research scientist at JD Explore Academy, Beijing, China. Previously, he was a senior researcher at Tencent AI Lab. He received his bachelor’s degree and Ph.D. from the School of Mathematics, South China University of Technology. His research interests include theory and algorithms for nonsmooth convex and nonconvex optimization, and their applications in trustworthy artificial intelligence, deep learning and reinforcement learning. He has published more than 100 papers in peer-reviewed top-tier journal papers (JMLR, IEEE TPAMI, IJCV, IEEE TSP, IEEE TIP, IEEE TKDE, etc.) and conference papers (ICML, NeurIPS, ICLR, CVPR, ICCV, etc.). He has also served as the senior program committees for AAAI 2022, AAAI 2024 and area chairs for ICML 2024, ICLR 2024, ICPR 2022, ICPR 2024.
沈力分别于2013年和2017年在华南理工大学数学学院获得理学学士学位和运筹学与控制论博士学位。目前就职于京东探索研究院,任算法科学家。在加入京东之前,他曾任腾讯AI Lab高级研究员。他的研究方向为大规模优化算法与理论,及其在可信人工智能,深度学习,强化学习中的应用。目前他在机器学习与人工智能领域的旗舰期刊 (JMLR, IEEE TPAMI, IJCV, IEEE TSP, IEEE TIP, IEEE TKDE等)和顶级会议(ICML, NeurIPS, ICLR, CVPR, ICCV等)发表论文100余篇。另外,他曾任AAAI 2022, AAAI 2024的高级程序委员会成员和ICML 2024, ICLR 2024, ICPR 2022, ICPR 2024的领域主席。