Mar 28, 2024 Thursday
Abstract:
In this presentation, we offer a comprehensive analysis of the application of deep neural networks over the past decade from the viewpoint of compressive data encoding and decoding. We propose that the primary goal of learning (or intelligence) is to acquire a compact and structured representation of the sensed data distribution. The quality of the final representation can be evaluated by a principled metric called the information gain, which is calculated by the (lossy) coding rates of the learned features. We argue that the unrolled iterative optimization of this objective provides a coherent white-box explanation for almost all deep neural networks that have been widely used in artificial intelligence practice, including ResNets and Transformers. We will demonstrate through theoretical and empirical evidence that deep networks that are mathematically interpretable, practically effective, and semantically meaningful are now attainable.
Time:
Mar 28, 2024 Thursday
11:00-11:50
Location:
Rm W1-101, GZ Campus
Online Zoom
Join Zoom at: https://hkust-gz-edu-cn.zoom.us/j/4236852791 OR 423 685 2791
Speaker Bio:
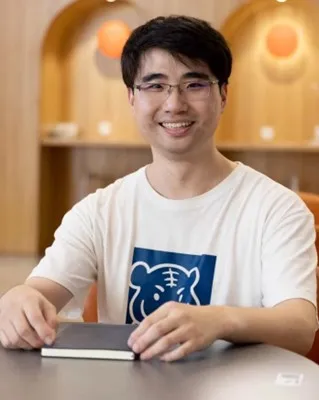
Weida WANG
PhD student, Tsinghua-Berkeley Shenzhen Institute
Weida Wang is a Ph.D. student at the Tsinghua-Berkeley Shenzhen Institute, where he is supervised by Professor Yi Ma and Professor Shao-Lun Huang. His research is centered on Information Theory and Multimodal Learning. Under the guidance of Professor Yi, his research group is dedicated to advancing autonomous intelligence through the development of transparent and consistent deep representation learning.