February 6, 2023 Monday
Abstract:
Transferability of deep neural networks (DNNs) refers to their ability to reuse knowledge (i.e., parameters) learned in one domain to solve problems in a different domain. There are two commonly held consensuses about the transferability of pre-trained DNNs: (1) the large domain gap between the source and target domains significantly reduces the transferability of pre-trained models, and (2) the lower layers (near the input) are more transferable than the higher layers (near the output). However, there has been no systematic exploration of how pre-trained parameters behave when they transfer to support these consensuses. In this work, we propose the first quantitative method to measure DNNs’ parameter transferability using optimization path length. By analyzing possible factors of transferability on twelve representative image datasets, we surprisingly find that the domain gap has a small effect on transferability, while downstream data diversity and data amount play more important roles. More importantly, we find that the middle layers of DNNs are the most transferable, rather than the lower layers as we previously thought. Our findings, which are validated by rich experimental analyses, challenge the previous consensuses about knowledge transfer in DNNs. They are promising to promote and innovate the development of this field.
Speaker Bio
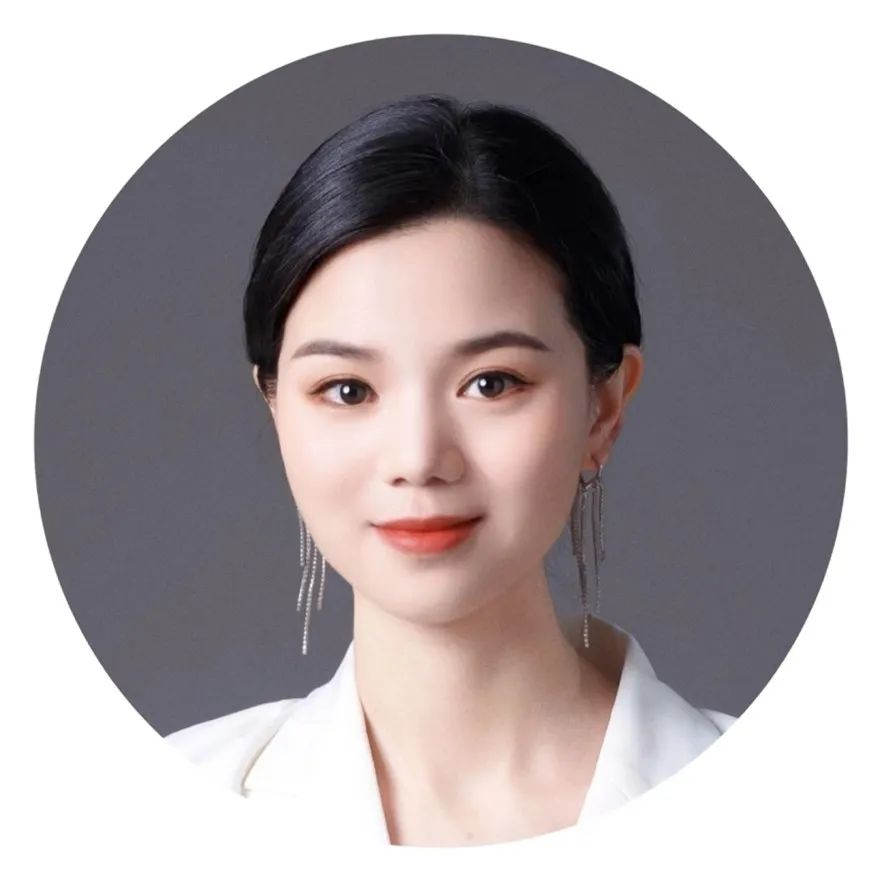
Dr. Li LIU
Assistant Professor, AI Thrust, INFH,HKUST(GZ)
Dr. Li Liu is an assistant professor at Artificial Intelligence Thrust, Information Hub, Hong Kong University of Science and Technology (Guangzhou). She obtained her Ph.D. degree in 2018 from Gipsa-lab, University Grenoble Alpes (UGA), Grenoble, France. Her main research interests include few-shot learning, AI Security and Transferability, and their applications in audio-visual speech recognition/generation and AI medical Imaging. As the first author or corresponding author, she has published about 30 papers in top journals and conferences in related fields, including IEEE Transactions on Pattern Analysis and Machine Intelligence, IEEE Transactions on Multimedia, NeurIPS, ECCV, MICCAI and ICASSP etc. In 2017, she won the French Sephora Berribi Scholarship for Female Scientists in Mathematics and Computers (totally four in the world, two in France and two in Israel). In the same year, she was awarded a scholarship for young researchers by the French Voice Association (AFCP). Her research has been funded by several scientific research projects, including the National Natural Science Foundation of China-Youth Fund, Guangdong Provincial Natural Science Foundation of Youth Science Fund, Shenzhen Excellent Science and Technology Innovation Talent Doctoral Entrepreneurship Project, Alibaba Innovation Research Fund and Tencent Technology Venture Philanthropy Program. In 2022, her two papers were selected as the Shenzhen Excellent Science and Technology Academic Papers.